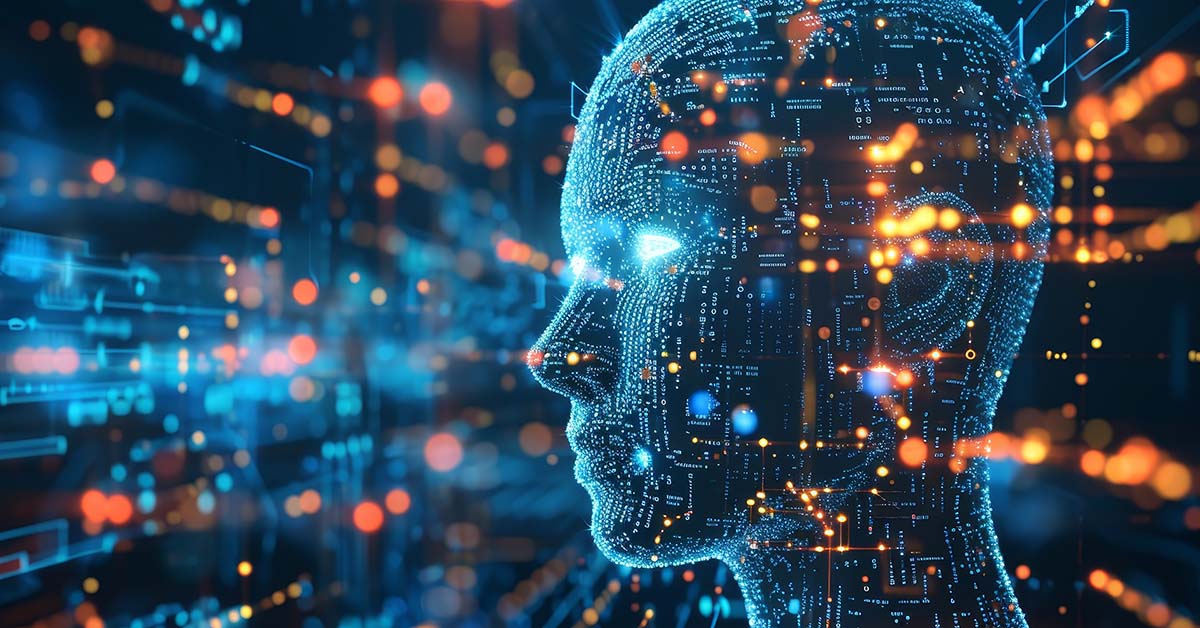
Contents
It's been ten years since we started our journey of building a threat intelligence platform, and a lot has changed since then. However, some underlying principles remain the same, and they should. Security is a serious matter, and how we apply technology to solve security problems needs to be done with very careful consideration of what is at stake.
Working in security means evaluating intelligence sources in a very short period of time, determining if there's a potential threat or risk, how severe we think it might be, escalating it, and determining how to respond. All of this needs to happen within minutes.
In recent years, the geopolitical landscape has become far more unstable and unpredictable than it has been in the last several decades. We have experienced extreme political divides, a surge in terrorism, armed conflicts, and a general sense of distrust in politics, governments, and the media.
Against this backdrop of divisiveness, our sources of information have gone from a few mainstream sources, such as news outlets and social media networks, to a broad range of niche sources that cater to different audiences, communities and political orientations. While it's hard to estimate, according to several sources, in 2014, we generated 12.5 zettabytes of data, and in 2024, we’re projected to generate 147 zettabytes of data. Regardless of what a zettabyte is (it’s a trillion gigabytes, in case you were wondering), the fact is that we are producing 12 times more data than ten years ago.
Technology has played a significant role in spreading false narratives, seeding division, reinforcing extreme views and breaking social dialogue, creating a more isolated society and increasing levels of loneliness and mental health challenges.
Over the last couple of years, we have seen mind-blowing progress in the domain of artificial intelligence (AI), allowing the general public to leverage AI in ways that were only available to academics and researchers before, with a level of refinement and accuracy that makes several use cases viable today, that were merely experimental not so long ago.
While many beneficial use cases for AI help improve our everyday lives, the ease of access has also led to bad actors leveraging AI for scams, extortion, fraud, and a host of other criminal activities. The ability to readily create credible deep fakes of images, video, or voice, as well as the ability to generate compelling and well-written text, has created a breeding ground for all sorts of potential threats.
This is the stage in which security analysts work today; there aren’t enough human resources to monitor all sources of information, let alone the volume of data being produced daily. Threat actors and threats are becoming more sophisticated and difficult to identify. We rely heavily on technology to do almost anything these days, and while software can be of great help to automate tasks that are repetitive and time-consuming, it can’t and shouldn’t ever replace human judgment.
In this context, AI can really help security analysts do their jobs more effectively. However, careful consideration needs to be given to how we do that to ensure we’re not letting a model make decisions that can impact human lives.
In recent years, AI has made incredible leaps in understanding context and producing predictions that are far more accurate than they were even five years ago. Despite this significant progress, AI models are not perfect and will always have a certain error margin.
How Can We Use AI Responsibly In Threat Intelligence?
Let's start by discussing the elements of open source threat intelligence (OSINT). The foundational aspect of OSINT is leveraging publicly available data, which is, at its core, the raw ingredient that we have to work with. Data can come in different forms: text, images, video, and audio, as well as transactional data like earthquakes, flight data, traffic, and other publicly available sources of intel.
Anyone who has worked in this space knows that open source data is far from clean and digestible. Humans create billions of new publicly available data points daily that can inform threat intelligence operations.
By searching and filtering through the available data using combinations of search terms and boolean logic, we can discard most of that data, leaving thousands of data points to evaluate. So by using these filtering strategies we have now boiled the ocean down to a lake. This is where AI comes in, and we can leverage it for a number of different purposes.
A fairly significant portion of the data that analysts are exposed to is duplicate (think about posts that get reposted thousands of times) or simply noise generated by bot accounts. AI can safely help identify content that is very similar, saving countless hours of work for analysts who would otherwise come across the same content over and over again.
Now, we’re left with content that is unique and relatively meaningful. We can again leverage AI to extract insights from that content that can help us determine if it's valuable or not. AI can help us infer elements like emotions, threat categories, severity, location and other metrics. This is where when we’re practicing responsible AI we need to draw a line in the sand in terms of how we leverage these extracted features.
An analyst can now filter or sort their remaining data by any combination of these features to help prioritize the content that is more likely to be actionable, allowing them to quickly review the data points that perhaps contain negative emotions, threats and that are in proximity to the people or assets that they are trying to keep safe.
We aren’t leveraging AI to do the analyst’s job, but we are rather enabling the analyst to fulfill their duty of care more efficiently and faster. The time it takes to identify, validate and respond to a threat is critical to the chances of successful prevention or mitigation.
We can also use these extracted features to identify trends over time, such as increased levels of negativity around a certain topic of location. While a trend is not a threat, it can be a leading indicator of increased risk.
In approaching AI responsibly, we ensure that at the end of the decision chain there is a human that is looking at the available insights and making a judgment call.
How Do We Build Ethical AI?
Ethical AI encompasses a set of principles and guidelines aimed at promoting fairness, transparency, accountability, and privacy in the development and deployment of AI systems.
Let’s go over some of the key considerations:
Fairness: AI algorithms should be designed to mitigate bias and discrimination, ensuring equitable treatment of individuals and groups across diverse demographics. In threat intelligence, fairness entails avoiding profiling or targeting based on sensitive attributes such as race, gender, religion, or nationality.
Transparency is essential for fostering trust and understanding in AI systems. AI systems can be highly complex and sometimes obscure, in terms of how they work and how they make decisions. It's critical that software vendors are able to explain how their AI and algorithms work and what elements are considered when these systems make predictions.
It is equally important to be transparent about any limitations or shortcomings that these systems inherently have. This allows the end user to understand what types of false positives or negatives can happen and how to deal with them. No AI system is perfect, but they are tremendously useful. By better understanding how they operate and what their limitations are, humans can rely on them to make better decisions.
Accountability involves holding developers, users, and stakeholders accountable for the ethical implications of AI technologies. Organizations should establish mechanisms for identifying and addressing instances of AI misuse, errors, or unintended consequences. In the context of threat intelligence, accountability is critical for ensuring responsible information sharing and preventing misuse of intelligence data.
Privacy protection is paramount in AI-driven threat intelligence and security solutions, as they often involve the processing of sensitive personal or organizational data. It is essential to implement robust privacy safeguards, such as data anonymization, encryption, and access controls, to prevent unauthorized access or disclosure of sensitive information. In addition to these safeguards, it's important to consider when it's safe to use a third-party state of the art model that is hosted on a vendor’s cloud, and when it's necessary to bring that processing in house to avoid sharing sensitive data with third parties.
AI Regulatory Frameworks
In 2016 the European Union published a regulatory framework for data protection and privacy that would come into force in 2018. GDPR has undoubtedly become the gold standard that other jurisdictions have followed to implement their own versions of privacy focused regulatory frameworks.
Once again, last month, the EU took the lead in approving a regulatory framework for Artificial Intelligence that sets the foundation for how we will start to regulate AI moving forward.
Rather than the somewhat extreme narratives that have been spun up in the last year around AI being an existential threat to humanity, the EU has focused on clearly defining what constitutes AI and then classifying these AI systems based on their level of risk.
As a general rule, The EU AI Act aims to classify and regulate AI applications based on their risk to cause harm. The classification categorizes the level of risk of these systems into four categories ranging from unacceptable risk all the way to minimal risk. As the word implies, applications that fall into the unacceptable category are banned. High-risk and limited risk applications are subject to a ranging set of controls related to security, transparency and quality.
Amongst the AI systems that have been identified as unacceptable or high risk by the EU framework are several that can impact law enforcement and security practitioners:
- Biometric categorization systems that attempt to categorize people according to highly sensitive traits, such as political orientation, religious or philosophical beliefs, sexual orientation, culture or race.
- “Real-time” biometric identification for law enforcement outside of a select set of use cases (targeted search for missing or abducted persons, imminent threat to life or safety/terrorism, or prosecution of a specific crime)
- Predictive policing
- Broad facial recognition/biometric scanning, clearly targeting technologies like Clearview.
Powerful general purpose AI models like ChatGPT and others have not been identified as high risk by the regulation, but their downstream use in applications that fall under the risk classification would be banned or regulated.
A number of jurisdictions have also been working on their own AI regulatory frameworks, like the US AI Bill of Rights, and similar initiatives in Canada and other countries. While this will add complexity to the work of data scientists and AI companies, it is a very welcome and much needed intervention to ensure that AI is being used and developed responsibly to minimize risk to individuals and groups.
What Lies Ahead For Security Practitioners In The Era Of AI?
We strongly believe that today more than ever, security practitioners play an essential role in mitigating risk for business operations and their assets, but more importantly, keeping people safe.
As described above, the risk landscape has become far more complex, and open source intelligence is more relevant than ever but is also harder to leverage.
Our vision is centred around the concept of extended intelligence, where AI isn’t viewed as a replacement for human intelligence, but rather as a tool to assist humans and expand their abilities to do an incredibly challenging job as effectively as possible.
As a co-founder of LifeRaft with 20+ years in the software industry, Eduardo leads the innovation team to advance our AI, data enrichments and data acquisition strategy.